DAGStat 2025: Statistics in the Times of AI
Jeremy Oguamalam participated in the 7th Joint Statistical Meeting of the Deutsche Arbeitsgemeinschaft Statistik.
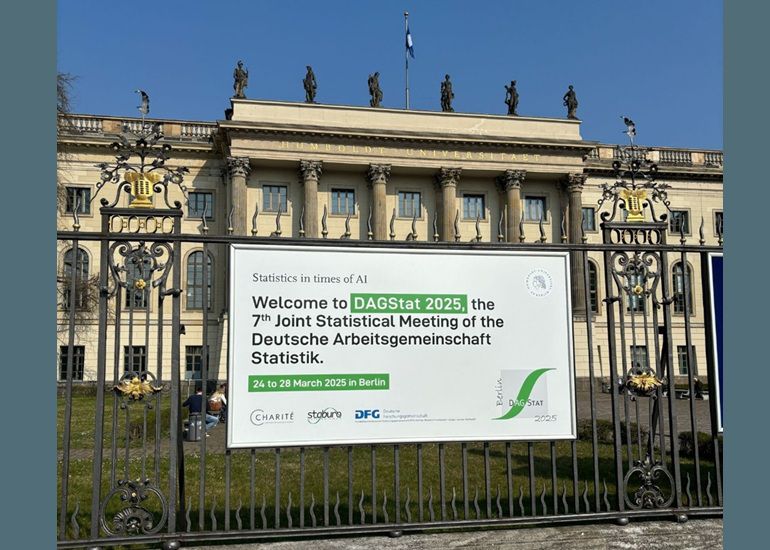
Jeremy Oguamalam participated in the 7th Joint Statistical Meeting of the Deutsche Arbeitsgemeinschaft Statistik (DAGStat 2025), held from March 24–28 at Humboldt Universität zu Berlin. Themed “Statistics in the Times of AI”, the conference brought together researchers from across disciplines to explore the evolving role of statistics in the age of artificial intelligence. Below is the abstract of the presented contribution, as published in the official Book of Abstracts (DAGStat2025_BoA.pdf):
This paper introduces a robust approach to functional principal component analysis (FPCA) for compositional data, particularly density functions. While recent papers have studied density data within the Bayes space framework, there has been limited focus on developing robust methods to effectively handle anomalous observations and large noise. To address this, we extend the Mahalanobis distance concept to Bayes spaces, proposing its regularized version that accounts for the constraints inherent in density data. Based on this extension, we introduce a new method, robust density principal component analysis (RDPCA), for more accurate estimation of functional principal components in the presence of outliers. The method’s performance is validated through simulations and real-world applications, showing its ability to improve covariance estimation and principal component analysis compared to traditional methods.